Invited Speakers
Joris Degroote
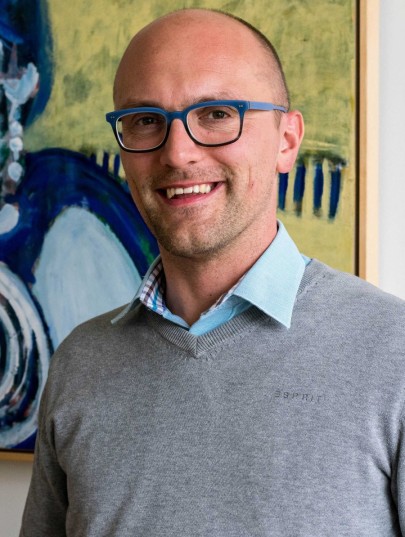
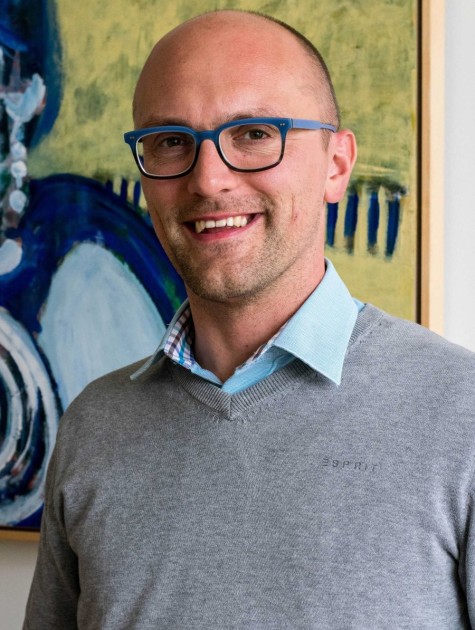
Ghent University, Ghent, Belgium
Title: Recent advances in quasi-Newton methods for partitioned simulation of fluid-structure interaction
Abstract: Quasi-Newton methods can be used in partitioned fluid-structure interaction simulations if there are coupling iterations between the solvers and the convergence needs to be stabilized and accelerated. Recently, it has been shown how surrogate models of the actual solvers can be included in these algorithms to further accelerate their convergence. This also led to the reformulation of several existing quasi-Newton methods in the generalized Broyden framework and to more emphasis on the necessity of linear scaling with the number of degrees of freedom on the coupling interface. Furthermore, numerical experiments have demonstrated that limiting the amount of work in each solver per coupling iteration can be beneficial for the total duration of the simulation, outweighing the increase in number of coupling iterations up to a certain point. Finally, the additional coupling tolerance can be avoided if some information about the convergence of the solvers is accessible to the coupling framework. Individually or combined, these recent evolutions can lead to a meaningful reduction in simulation time.
Joris Degroote (1),(3); Nicolas Delaissé (1); Thomas Spenke (2) and Norbert Hosters (2)
(1) Department of Electromechanical, Systems and Metal Engineering, Faculty of Engineering and Architecture, Ghent University – Ghent, Belgium
(2) Chair for Computational Analysis of Technical Systems (CATS), Center for Simulation and Data Science (JARA-CSD), RWTH Aachen University – Aachen, Germany
(3) Core Lab MIRO, Flanders Make – Ghent, Belgium
Somdatta Goswami
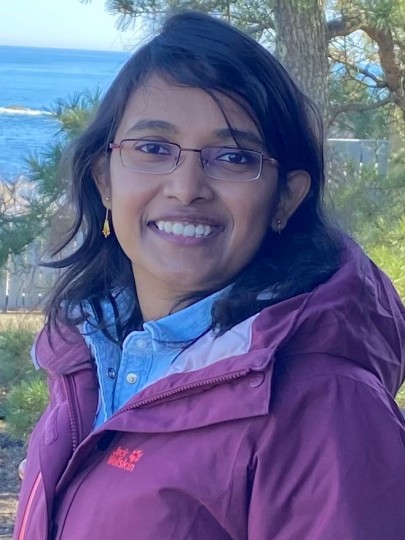
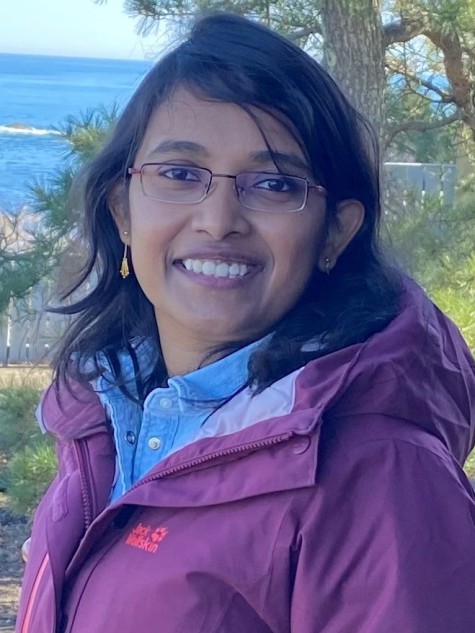
Johns Hopkins University, Baltimore (MD), USA
Title: Employing Machine Learning Approaches to solve PDEs in “Mechanics” within Big-data Regime
Abstract: A new paradigm in scientific research has been established with the integration of data-driven and physics-informed methodologies in the domain of deep learning, and it is certain to have an impact on all areas of science and engineering. This field, popularly termed “scientific machine learning,” relies on an over-parametrized deep learning model trained with (or without) high-fidelity data (simulated or experimental) to be able to generalize the solution field across multiple input instances. The neural operator framework fulfills this promise by learning the mapping between infinite dimensional functional spaces. The application of neural operators’ techniques within the context of operator regression to resolve efficiently and accurately time-dependent and -independent PDEs in mechanics will be the major focus of this presentation. The approaches' extrapolation ability, accuracy, and computing efficiency when integrated with traditional numerical solvers will be discussed.
Angelika Humbert
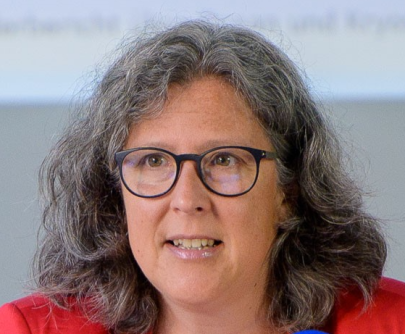
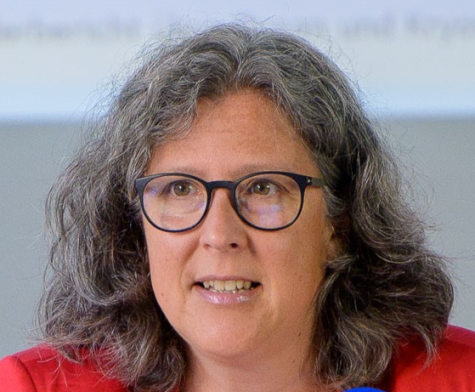
Alfred Wegener Institute, Helmholtz Centre for Polar and Marine Research, Bremerhaven and University of Bremen, Bremen, Germany
Title: Multi-physics simulations of the Greenland Ice Sheet – approaches and challenges in tackling a complex system
David Keyes
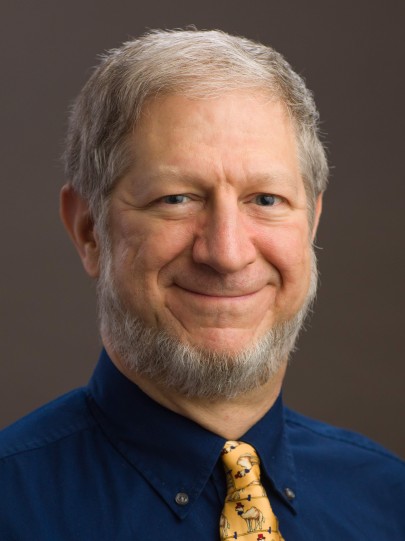
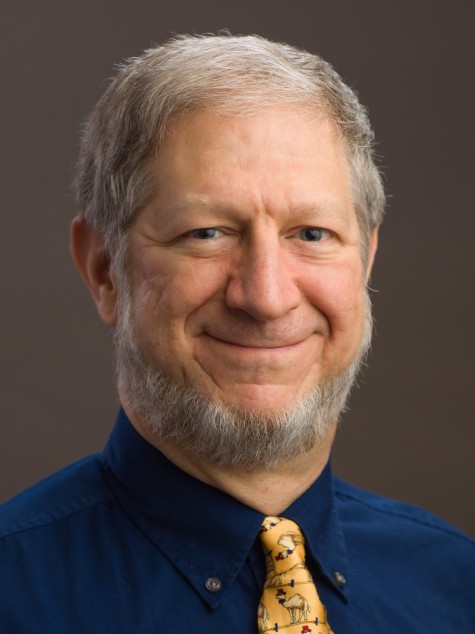
KAUST, Thuwal, Kingdom of Saudi Arabia
Title: Efficient Computation through Tuned Approximation
Abstract: Numerical software is being reinvented to provide opportunities to tune dynamically the accuracy of computation to the requirements of the application, resulting in savings of memory, time, and energy. Floating point computation in science and engineering has a history of “oversolving” relative to expectations for many models. So often are real datatypes defaulted to double precision that GPUs did not gain wide acceptance until they provided in hardware operations not required in their original domain of graphics. However, computational science is now reverting to employ lower precision arithmetic where possible. Many matrix operations considered at a blockwise level allow for lower precision and many blocks can be approximated with low rank near equivalents. This leads to smaller memory footprint, which implies higher residency on memory hierarchies, leading in turn to less time and energy spent on data copying, which may even dwarf the savings from fewer and cheaper flops. We provide examples from several application domains, including a look at campaigns in geospatial statistics, seismic processing, genome wide association studies, and climate emulation that earned Gordon Bell Prize finalist status in 2022, 2023, and 2024.
Stefan Turek
TU Dortmund, Dortmund, Germany
Title: The Future of CFD Simulations (from a numerical & computational perspective) – Faster and more reliable predictions and new benchmark concepts are needed to compete with AI
Abstract: The main aim of this talk is to discuss how modern High Performance Computing (HPC) techniques regarding massively parallel hardware with millions of cores together with very fast, but lower precision accelerator hardware can be applied to numerical simulations of PDEs so that a much higher computational, numerical and hence energy efficiency can be obtained. Here, as prototypical extreme-scale PDE-based applications, we concentrate on nonstationary flow simulations with hundreds of millions or even billions of spatial unknowns in long-time computations with many thousands up to millions of time steps. For the expected huge computational resources in the coming exascale era, such type of spatially discretized problems which typically are treated sequentially in time, that means one time after the other, are often too small to exploit adequately the huge number of compute nodes, resp., cores so that further parallelism, for instance w.r.t. time, might get necessary. In this context, we discuss how “parallel-in-space & global-in-time” Newton-Krylov Multigrid approaches can be designed which allow a much higher degree of parallelism. Moreover, to exploit current accelerator hardware in lower precision (for instance, GPUs from NVIDIA built for AI applications), we discuss the concept of “prehandling” (in contrast to “preconditioning”) of the corresponding ill-conditioned systems of equations, for instance arising from Poisson-like problems. Here, we assume a transformation into an equivalent linear system with similar sparsity but with much lower condition numbers so that the use of lower precision hardware gets feasible. In our talk, we provide for both aspects numerical results as “proof-of-concept” and discuss the challenges, particularly for incompressible flow problems, also in view of comparisons with AI predictions.